Individual Participant Data Meta-Analysis: A Handbook for Healthcare Research
​Content overview
​​
Split into five parts (containing 18 chapters & over 500 pages), the book takes the reader through the journey from initiating and planning IPD projects to obtaining, checking, and meta-analysing IPD, and appraising and reporting findings.
The book initially focuses on the synthesis of IPD from randomised trials to evaluate treatment effects, including the evaluation of participant-level effect modifiers (treatment-covariate interactions). Detailed extension is then made to specialist topics such as diagnostic test accuracy, prognostic factors, risk prediction models, and advanced statistical topics such as multivariate and network meta-analysis, power calculations, and missing data.
​
Available to purchase from about £60.00 at Amazon & Wiley
​
Table of contents​
​
Acknowledgements xxiii
1 Individual Participant Data Meta-analysis for Healthcare Research 1
Richard D. Riley, Lesley A. Stewart, and Jayne F. Tierney
1.1 Introduction 1
1.2 What Is IPD and How Does It Differ from Aggregate Data? 1
1.3 IPD Meta-analysis: A New Era for Evidence Synthesis 2
1.4 Scope of This Book and Intended Audience 2
​
Part I Rationale, Planning, and Conduct 7
2 Rationale for Embarking on an IPD Meta-analysis Project 9
Jayne F. Tierney, Richard D. Riley, Catrin Tudur Smith, Mike Clarke, and Lesley A. Stewart
2.1 Introduction 9
2.2 How Does the Research Process Differ for IPD and Aggregate Data Meta-analysis Projects? 10
2.2.1 The Research Aims 10
2.2.2 The process and methods 10
2.3 What Are the Potential Advantages of an IPD Meta-analysis Project? 11
2.4 What Are the Potential Challenges of an IPD Meta-Analysis Project? 14
2.5 Empirical Evidence of Differences between Results of IPD and Aggregate Data Meta-analysis Projects 14
2.6 Guidance for Deciding When IPD Meta-analysis Projects Are Needed to Evaluate Treatment Effects from Randomised Trials 15
2.6.1 Are IPD Needed to Tackle the Research Question? 15
2.6.2 Are IPD Needed to Improve the Completeness and Uniformity of Outcomes and Participant-level Covariates? 17
2.6.3 Are IPD Needed to Improve the Information Size? 17
2.6.4 Are IPD Needed to Improve the Quality of Analysis? 18
2.7 Concluding Remarks 19
​
3 Planning and Initiating an IPD Meta-analysis Project 21
Lesley A. Stewart, Richard D. Riley, and Jayne F. Tierney
3.1 Introduction 22
3.2 Organisational Approach 22
3.2.1 Collaborative IPD Meta-analysis Project 22
3.2.2 IPD Meta-analysis Projects Using Data Repositories or Data-sharing Platforms 24
3.3 Developing a Project Scope 26
3.4 Assessing Feasibility and ‘In Principle’ Support and Collaboration 26
3.5 Establishing a Team with the Right Skills 29
3.6 Advisory and Governance Functions 30
3.7 Estimating How Long the Project Will Take 31
3.8 Estimating the Resources Required 33
3.9 Obtaining Funding 38
3.10 Obtaining Ethical Approval 39
3.11 Data-sharing Agreement 41
3.12 Additional Planning for Prospective Meta-analysis Projects 41
3.13 Concluding Remarks 43
​
4 Running an IPD Meta-analysis Project: From Developing the Protocol to Preparing Data for Meta-analysis 45
Jayne F. Tierney, Richard D. Riley, Larysa H.M. Rydzewska, and Lesley A. Stewart
4.1 Introduction 46
4.2 Preparing to Collect IPD 46
4.2.1 Defining the Objectives and Eligibility Criteria 46
4.2.2 Developing the Protocol for an IPD Meta-analysis Project 49
4.2.3 Identifying and Screening Potentially Eligible Trials 51
4.2.4 Deciding Which Information Is Needed to Summarise Trial Characteristics 51
4.2.5 Deciding How Much IPD Are Needed 52
4.2.6 Deciding Which Variables Are Needed in the IPD 52
4.2.7 Developing a Data Dictionary for the IPD 55
4.3 Initiating and Maintaining Collaboration 57
4.4 Obtaining IPD 59
4.4.1 Ensuring That IPD Are De-identified 59
4.4.2 Providing Data Transfer Guidance 60
4.4.3 Transferring trial IPD securely 61
4.4.4 Storing Trial IPD Securely 61
4.4.5 Making Best Use of IPD from Repositories 61
4.5 Checking and Harmonising Incoming IPD 62
4.5.1 The Process and Principles 63
4.5.2 Initial Checking of IPD for Each Trial 63
4.5.3 Harmonising IPD across Trials 64
4.5.4 Checking the Validity, Range and Consistency of Variables 65
4.6 Checking the IPD to Inform Risk of Bias Assessments 66
4.6.1 The Randomisation Process 68
4.6.2 Deviations from the Intended Interventions 71
4.6.3 Missing Outcome Data 73
4.6.4 Measurement of the Outcome 74
4.7 Assessing and Presenting the Overall Quality of a Trial 76
4.8 Verification of Finalised Trial IPD 77
4.9 Merging IPD Ready for Meta-analysis 77
4.10 Concluding Remarks 80
Part I References 81
Part II Fundamental Statistical Methods and Principles 87
5 The Two-stage Approach to IPD Meta-analysis 89
Richard D. Riley, Thomas P.A. Debray, Tim P. Morris, and Dan Jackson
5.1 Introduction 90
5.2 First Stage of a Two-stage IPD Meta-analysis 90
5.2.1 General Format of Regression Models to Use in the First Stage 92
5.2.2 Estimation of Regression Models Applied in the First Stage 92
5.2.3 Regression for Different Outcome Types 94
5.2.3.1 Continuous Outcomes 94
5.2.3.2 Binary Outcomes 98
5.2.3.3 Ordinal and Multinomial Outcomes 99
5.2.3.4 Count and Incidence Rate Outcomes 100
5.2.3.5 Time-to-Event Outcomes 101
5.2.4 Adjustment for Prognostic Factors 102
5.2.5 Dealing with Other Trial Designs and Missing Data 103
5.3 Second Stage of a Two-stage IPD Meta-analysis 106
5.3.1 Meta-analysis Assuming a Common Treatment Effect 106
5.3.2 Meta-analysis Assuming Random Treatment Effects 107
5.3.3 Forest Plots and Percentage Trial Weights 110
5.3.4 Heterogeneity Measures and Statistics 110
5.3.5 Alternative Weighting Schemes 112
5.3.6 Frequentist Estimation of the Between-Trial Variance of Treatment Effect 113
5.3.7 Deriving Confidence Intervals for the Summary Treatment Effect 113
5.3.8 Bayesian Estimation Approaches 115
5.3.8.1 An Introduction to Bayes’ Theorem and Bayesian Inference 115
5.3.8.2 Using a Bayesian Meta-Analysis Model in the Second Stage 115
5.3.8.3 Applied Example 117
5.3.9 Interpretation of Summary Effects from Meta-analysis 118
5.3.10 Prediction Interval for the Treatment Effect in a New Trial 118
5.4 Meta-regression and Subgroup Analyses 120
5.5 The ipdmetan Software Package 121
5.6 Combining IPD with Aggregate Data from non-IPD Trials 124
5.7 Concluding Remarks 125
​
6 The One-stage Approach to IPD Meta-analysis 127
Richard D. Riley and Thomas P.A. Debray 127
6.1 Introduction 128
6.2 One-stage IPD Meta-analysis Models Using Generalised Linear Mixed Models 129
6.2.1 Basic Statistical Framework of One-stage Models Using GLMMs 129
6.2.1.1 Continuous Outcomes 130
6.2.1.2 Binary Outcomes 130
6.2.1.3 Ordinal and Multinomial Outcomes 135
6.2.1.4 Count and Incidence Rate Outcomes 136
6.2.2 Specifying Parameters as Either Common, Stratified, or Random 136
6.2.3 Accounting for Clustering of Participants within Trials 139
6.2.3.1 Examples 141
6.2.4 Choice of Stratified Intercept or Random Intercepts 141
6.2.4.1 Findings from Simulation Studies 142
6.2.4.2 Our Preference for Using a Stratified Intercept 142
6.2.4.3 Allowing for Correlation between Random Effects on Intercept and Treatment Effect 143
6.2.5 Stratified or Common Residual Variances 144
6.2.6 Adjustment for Prognostic Factors 145
6.2.7 Inclusion of Trial-level Covariates 145
6.2.8 Estimation of One-stage IPD Meta-analysis Models Using GLMMs 146
6.2.8.1 Software for Fitting One-stage Models 146
6.2.8.2 ML Estimation and Downward Bias in Between-trial Variance Estimates 146
6.2.8.3 Trial-specific Centering of Variables to Improve ML Estimation of One-stage Models with a Stratified Intercept 147
6.2.8.4 REML Estimation 147
6.2.8.5 Deriving Confidence Intervals for ParametersPpost-estimation 149
6.2.8.6 Prediction Intervals 151
6.2.8.7 Derivation of Percentage Trial Weights 151
6.2.8.8 Bayesian Estimation for One-stage Models 151
6.2.9 A Summary of Recommendations 152
6.3 One-stage Models for Time-to-event Outcomes 152
6.3.1 Cox Proportional Hazard Framework 152
6.3.1.1 Stratifying Using Proportional Baseline Hazards and Frailty Models 152
6.3.1.2 Stratifying Baseline Hazards without Assuming Proportionality 154
6.3.1.3 Comparison of Approaches 154
6.3.1.4 Estimation Methods 154
6.3.1.5 Example 156
6.3.2 Fully Parametric Approaches 157
6.3.3 Extension to Time-varying Hazard Ratios and Joint Models 157
6.4 One-stage Models Combining Different Sources of Evidence 159
6.4.1 Combining IPD Trials with Partially Reconstructed IPD from Non-IPD Trials 159
6.4.2 Combining IPD and Aggregate Data Using Hierarchical Related Regression 160
6.4.3 Combining IPD from Parallel Group, Cluster and Cross-over Trials 161
6.5 Reporting of One-stage Models in Protocols and Publications 162
6.6 Concluding Remarks 162
​
7 Using IPD Meta-analysis to Examine Interactions between Treatment Effect and Participant-level Covariates 163
Richard D. Riley and David J. Fisher
7.1 Introduction 164
7.2 Meta-regression and Its Limitations 166
7.2.1 Meta-regression of Aggregated Participant-level Covariates 166
7.2.2 Low Power and Aggregation Bias 166
7.2.3 Empirical Evidence of the Difference Between Using Across-trial and Within-trial Information to Estimate Treatment-covariate Interactions 167
7.3 Two-stage IPD Meta-analysis to Estimate Treatment-covariate Interactions 168
7.3.1 The Two-stage Approach 168
7.3.2 Applied Example: Is the Effect of Anti-hypertensive Treatment Different for Males and Females? 170
7.3.3 Do Not Quantify Interactions by Comparing Meta-analysis Results for Subgroups 171
7.4 The One-stage Approach 174
7.4.1 Merging Within-trial and Across-trial Information 174
7.4.2 Separating Within-trial and Across-trial Information 175
7.4.2.1 Approach (i) for a One-stage Survival Model: Center the Covariate and Include the Covariate Mean 175
7.4.2.2 Approach (ii) for a One-stage Survival Model: Stratify All Nuisance Parameters by Trial 176
7.4.2.3 Approaches (i) and (ii) for Continuous and Binary Outcomes 176
7.4.2.4 Comparison of Approaches (i) and (ii) 177
7.4.3 Applied Examples 177
7.4.3.1 Is Age an Effect Modifier for Epilepsy Treatment? 177
7.4.3.2 Is the Effect of an Early Support Hospital Discharge Modified by Having a Carer Present? 178
7.4.4 Coding of the Treatment Covariate and Adjustment for Other Covariates 178
7.4.4.1 Example 180
7.4.5 Estimating the Aggregation Bias Directly 180
7.4.6 Reporting Summary Treatment Effects for Subgroups after Adjusting for Aggregation Bias 180
7.5 Combining IPD and non-IPD Trials 181
7.5.1 Can We Recover Interaction Estimates from non-IPD Trials? 181
7.5.2 How to Incorporate Interaction Estimates from non-IPD Trials in an IPD Metaanalysis 182
7.6 Handling of Continuous Covariates 184
7.6.1 Do Not Categorise Continuous Covariates 184
7.6.2 Interactions May Be Non-linear 185
7.6.2.1 Rationale and an Example 185
7.6.2.2 Two-stage Multivariate IPD Meta-analysis for Summarising Non-linear Interactions 186
7.6.2.3 One-stage IPD Meta-analysis for Summarising Non-linear Interactions 190
7.7 Handling of Categorical or Ordinal Covariates 191
7.8 Misconceptions and Cautions 191
7.8.1 Genuine Treatment-covariate Interactions Are Rare 191
7.8.2 Interactions May Depend on the Scale of Analysis 192
7.8.3 Measurement Error May Impact Treatment-covariate Interactions 193
7.8.4 Even without Treatment-covariate Interactions, the Treatment Effect on Absolute Risk May Differ across Participants 193
7.8.5 Between-trial Heterogeneity in Treatment Effect Should Not Be Used to Guide Whether Treatment-covariate Interactions Exist at the Participant Level 194
7.9 Is My Identified Treatment-covariate Interaction Genuine? 195
7.10 Reporting of Analyses of Treatment-covariate Interactions 196
7.11 Can We Predict a New Patient’s Treatment Effect? 196
7.11.1 Linking Predictions to Clinical Decision Making 198
7.12 Concluding Remarks 198
​
8 One-stage versus Two-stage Approach to IPD Meta-analysis: Differences and Recommendations 199
Richard D. Riley, Danielle L. Burke, and Tim Morris
8.1 Introduction 200
8.2 One-stage and Two-stage Approaches Usually Give Similar Results 200
8.2.1 Evidence to Support Similarity of One-stage and Two-stage IPD Meta-analysis Results 200
8.2.2 Examples 202
8.2.3 Some Claims in Favour of the One-stage Approach Are Misleading 203
8.3 Ten Key Reasons Why One-stage and Two-stage Approaches May Give Different Results 203
8.3.1 Reason I: Exact One-stage Likelihood When Most Trials Are Small 204
8.3.2 Reason II: How Clustering of Participants Within Trials Is Modelled 207
8.3.3 Reason III: Coding of the Treatment Variable in One-stage Models Fitting with ML Estimation 208
8.3.4 Reason IV: Different Estimation Methods for τ2 210
8.3.5 Reason V: Specification of Prognostic Factor and Adjustment Terms 210
8.3.6 Reason VI: Specification of the Residual Variances 212
8.3.7 Reason VI: Choice of Common Effect or Random Effects for the Parameter of Interest 213
8.3.8 Reason VIII: Derivation of Confidence Intervals 213
8.3.9 Reason IX: Accounting for Correlation Amongst Multiple Outcomes or Time-points 214
8.3.10 Reason X: Aggregation Bias for Treatment Covariate Interactions 215
8.3.11 Other Potential Causes 215
8.4 Recommendations and Guidance 216
8.5 Concluding Remarks 217
Part II References 219
​
Part III Critical Appraisal and Dissemination 237
9 Examining the Potential for Bias in IPD Meta-analysis Results 239
Richard D. Riley, Jayne F. Tierney, and Lesley A. Stewart
9.1 Introduction 240
9.2 Publication and Reporting Biases of Trials 240
9.2.1 Impact on IPD Meta-analysis Results 240
9.2.2 Examining Small-study Effects Using Funnel Plots 241
9.2.3 Small-study Effects May Arise Due to the Factors Causing Heterogeneity 243
9.3 Biased Availability of the IPD from Trials 244
9.3.1 Examining the Impact of Availability Bias 245
9.3.2 Example: IPD Meta-analysis Examining High-dose Chemotherapy for the Treatment of Non-Hodgkin Lymphoma 246
9.4 Trial Quality (risk of bias) 247
9.5 Other Potential Biases Affecting IPD Meta-analysis Results 248
9.5.1 Trial Selection Bias 248
9.5.2 Selective Outcome Availability 250
9.5.3 Use of Inappropriate Methods by the IPD Meta-analysis Research Team 250
9.6 Concluding Remarks 251
​
10 Reporting and Dissemination of IPD Meta-analyses 253
Lesley A. Stewart, Richard D. Riley, and Jayne F. Tierney
10.1 Introduction 253
10.2 Reporting IPD Meta-analysis Projects in Academic Reports 254
10.2.1 PRISMA-IPD Title and Abstract Sections 255
10.2.2 PRISMA-IPD Introduction Section 259
10.2.3 PRISMA-IPD Methods Section 259
10.2.4 PRISMA-IPD Results Section 262
10.2.5 PRISMA-IPD Discussion and Funding Sections 266
10.3 Additional Means of Disseminating Findings 266
10.3.1 Key Audiences 267
10.3.1.1 The IPD Collaborative Group 267
10.3.1.2 Patient and Public Audiences 267
10.3.1.3 Guideline Developers 268
10.3.2 Communication Channels 268
10.3.2.1 Evidence Summaries and Policy Briefings 268
10.3.2.2 Press Releases 268
10.3.2.3 Social Media 270
10.4 Concluding Remarks 270
​
11 A Tool for the Critical Appraisal of IPD Meta-analysis Projects (CheckMAP) 271
Jayne F. Tierney, Lesley A. Stewart, Claire L. Vale, and Richard D. Riley
11.1 Introduction 271
11.2 The CheckMAP Tool 272
11.3 Was the IPD Meta-analysis Project Done within a Systematic Review Framework? 272
11.4 Were the IPD Meta-analysis Project Methods Pre-specified in a Publicly Available Protocol? 274
11.5 Did the IPD Meta-analysis Project Have a Clear Research Question Qualified by Explicit Eligibility Criteria? 276
11.6 Did the IPD Meta-analysis Project Have a Systematic and Comprehensive Search Strategy? 276
11.7 Was the Approach to Data Collection Consistent and Thorough? 277
11.8 Were IPD Obtained from Most Eligible Trials and Their Participants? 277
11.9 Was the Validity of the IPD Checked for Each Trial? 278
11.10 Was the Risk of Bias Assessed for Each Trial and Its Associated IPD? 27811.10.1 Was the Randomisation Process Checked Based on IPD? 278
11.10.2 Were the IPD Checked to Ensure That All (or Most) Randomised Participants Were Included? 279
11.10.3 Were All Important Outcomes Included in the IPD? 279
11.10.4 Were the Outcomes Measured/Defined Appropriately? 279
11.10.5 Was the Quality of Outcome Data Checked? 280
11.11 Were the Methods of Meta-analysis Appropriate? 280
11.11.1 Were the Analyses Pre-specified in Detail and the Key Estimands Defined? 280
11.11.2 Were the Methods of Summarising the Overall Effects of Treatments Appropriate? 281
11.11.3 Were the Methods of Assessing whether Effects of Treatments Varied by Trial-level Characteristics Appropriate? 281
11.11.4 Were the Methods of Assessing whether Effects of Treatments Varied by Participant-level Characteristics Appropriate? 282
11.11.5 Was the Robustness of Conclusions Checked Using Relevant Sensitivity or Other Analyses? 282
11.11.6 Did the IPD Meta-analysis Project’s Report Cover the Items Described in PRISMAIPD? 282
11.12 Concluding Remarks 283
Part III References 285
​
Part IV Special Topics in Statistics 291
​
12 Power Calculations for Planning an IPD Meta-analysis 293
Richard D. Riley and Joie Ensor
12.1 Introduction 294
12.1.1 Rationale for Power Calculations in an IPD Meta-analysis 294
12.1.2 Premise for This Chapter 294
12.2 Motivating Example: Power of a Planned IPD Meta-analysis of Trials of Interventions to Reduce Weight Gain in Pregnant Women 295
12.2.1 Background 295
12.2.2 What Is the Power to Detect a Treatment-BMI Interaction? 295
12.2.3 Power of an IPD Meta-analysis to Detect a Treatment-covariate Interaction for a Continuous Outcome 295
12.2.4 Closed-form Solutions 296
12.2.4.1 Application to the i-WIP Example 298
12.2.5 Simulation-based Power Calculations for a Two-stage IPD Meta-analysis 299
12.2.5.1 Application to the i-WIP Example 300
12.2.6 Power Results Naively Assuming the IPD All Come from a Single Trial 301
12.3 The Contribution of Individual Trials Toward Power 301
12.3.1 Contribution According to Sample Size 301
12.3.2 Contribution According to Covariate and Outcome Variability 302
12.4 The Impact of Model Assumptions on Power 302
12.4.1 Impact of Allowing for Heterogeneity in the Interaction 302
12.4.2 Impact of Wrongly Modelling BMI as a Binary Variable 304
12.4.3 Impact of Adjusting for Additional Covariates 304
12.5 Extensions 305
12.5.1 Power Calculations for Binary and Time-to-event Outcomes 305
12.5.2 Simulation Using a One-stage IPD Meta-analysis Approach 306
12.5.3 Examining the Potential Precision of IPD Meta-analysis Results 307
12.5.4 Estimating the Power of a New Trial Conditional on IPD Meta-analysis Results 307
12.6 Concluding Remarks 309
​
13 Multivariate Meta-analysis Using IPD 311
Richard D. Riley, Dan Jackson, and Ian R. White
13.1 Introduction 312
13.2 General Two-stage Approach for Multivariate IPD Meta-analysis 314
13.2.1 First-stage Analyses 315
13.2.1.1 Obtaining Treatment Effect Estimates and Their Variances for Continuous Outcomes 315
13.2.1.2 Obtaining Within-trial Correlations Directly or via Bootstrapping for Continuous Outcomes 316
13.2.1.3 Extension to Binary, Time-to-event and Mixed Outcomes 317
13.2.2 Second-stage Analysis: Multivariate Meta-analysis Model 319
13.2.2.1 Multivariate Model Structure 320
13.2.2.2 Dealing with Missing Outcomes 320
13.2.2.3 Frequentist Estimation of the Multivariate Model 321
13.2.2.4 Bayesian Estimation of the Multivariate Model 322
13.2.2.5 Joint Inferences and Predictions 322
13.2.2.6 Alternative Specifications for the Between-trial Variance Matrix with Missing Outcomes 323
13.2.2.7 Combining IPD and non-IPD Trials 323
13.2.3 Useful Measures to Accompany Multivariate Meta-analysis Results 324
13.2.3.1 Heterogeneity Measures 324
13.2.3.2 Percentage Trial Weights 325
13.2.3.3 The Efficiency (E) and Borrowing of Strength (BoS) Statistics 325
13.2.4 Understanding the Impact of Correlation and Borrowing of Strength 326
13.2.4.1 Anticipating the Value of BoS When Assuming Common Treatment Effects 326
13.2.4.2 BoS When Assuming Random Treatment Effects 327
13.2.4.3 How the Borrowing of Strength Impacts upon the Summary Meta-analysis Estimates 327
13.2.4.4 How the Correlation Impacts upon Joint Inferences across Outcomes 328
13.2.5 Software 328
13.3 Application to an IPD Meta-analysis of Anti-hypertensive Trials 329
13.3.1 Bivariate Meta-analysis of SBP and DBP 329
13.3.1.1 First-stage Results 329
13.3.1.2 Second-stage Results 329
13.3.1.3 Predictive Inferences 331
13.3.2 Bivariate Meta-analysis of CVD and Stroke 332
13.3.3 Multivariate Meta-analysis of SBP, DBP, CVD and Stroke 332
13.4 Extension to Multivariate Meta-regression 333
13.5 Potential Limitations of Multivariate Meta-analysis 334
13.5.1 The Benefits of a Multivariate Meta-analysis for Each Outcome Are Often Small 335
13.5.2 Model Specification and Estimation Is Non-trivial 335
13.5.3 Benefits Arise under Assumptions 335
13.6 One-stage Multivariate IPD Meta-analysis Applications 337
13.6.1 Summary Treatment Effects 337
13.6.1.1 Applied Example 337
13.6.2 Multiple Treatment-covariate Interactions 337
13.6.2.1 Applied Example 339
13.6.3 Multinomial Outcomes 339
13.7 Special Applications of Multivariate Meta-analysis 340
13.7.1 Longitudinal Data and Multiple Time-points 340
13.7.1.1 Applied Example 341
13.7.1.2 Extensions 342
13.7.2 Surrogate Outcomes 342
13.7.3 Development of Multi-parameter Models for Dose Response and Prediction 344
13.7.4 Test Accuracy 345
13.7.5 Treatment-covariate Interactions 345
13.7.5.1 Non-linear Trends 345
13.7.5.2 Multiple Treatment-covariate Interactions 345
13.8 Concluding Remarks 346
​
14 Network Meta-analysis Using IPD 347
Richard D. Riley, David M. Phillippo, and Sofia Dias
14.1 Introduction 348
14.2 Rationale and Assumptions for Network Meta-analysis 348
14.3 Network Meta-analysis Models Assuming Consistency 350
14.3.1 A Two-stage Approach 350
14.3.2 A One-stage Approach 351
14.3.3 Summary Results after a Network Meta-analysis 352
14.3.4 Example: Comparison of Eight Thrombolytic Treatments after Acute Myocardial Infarction 352
14.3.4.1 Two-stage Approach 353
14.3.4.2 One-stage Approach 357
14.4 Ranking Treatments 357
14.5 How Do We Examine Inconsistency between Direct and Indirect Evidence? 359
14.6 Benefits of IPD for Network Meta-analysis 361
14.6.1 Benefit 1: Examining and Plotting Distributions of Covariates across Trials Providing Different Comparisons 361
14.6.2 Benefit 2: Adjusting for Prognostic Factors to Improve Consistency and Reduce Heterogeneity 361
14.6.3 Benefit 3: Including Treatment-covariate Interactions 362
14.6.4 Benefit 4: Multiple Outcomes 365
14.7 Combining IPD and Aggregate Data in Network Meta-analysis 365
14.7.1 Multilevel Network Meta-regression 367
14.7.2 Example: Treatments to Reduce Plaque Psoriasis 369
14.8 Further Topics 370
14.8.1 Accounting for Dose and Class 370
14.8.2 Inclusion of ‘Real-world’ Evidence 372
14.8.3 Cumulative Network Meta-analysis 372
14.8.4 Quality Assessment and Reporting 372
14.9 Concluding Remarks 372
Part IV References 375
​
Part V Diagnosis, Prognosis and Prediction 387
​
15 IPD Meta-analysis for Test Accuracy Research 389
Richard D. Riley, Brooke Levis, and Yemisi Takwoingi 389
15.1 Introduction 390
15.1.1 Meta-analysis of Test Accuracy Studies 390
15.1.2 The Need for IPD 391
15.1.3 Scope of This Chapter 394
15.2 Motivating Example: Diagnosis of Fever in Children Using Ear Temperature 394
15.3 Key Steps Involved in an IPD Meta-analysis of Test Accuracy Studies 397
15.3.1 Defining the Research Objectives 397
15.3.2 Searching for Studies with Eligible IPD 397
15.3.3 Extracting Key Study Characteristics and Information 398
15.3.4 Evaluating Risk of Bias of Eligible Studies 398
15.3.5 Obtaining, Cleaning and Harmonising IPD 401
15.3.6 Undertaking IPD Meta-analysis to Summarise Test Accuracy at a Particular Threshold 401
15.3.6.1 Bivariate IPD Meta-analysis to Summarise Sensitivity and Specificity 401
15.3.6.2 Examining and Summarising Heterogeneity 402
15.3.6.3 Combining IPD and non-IPD Studies 403
15.3.6.4 Application to the Fever Example 403
15.3.6.5 Bivariate Meta-analysis of PPV and NPV 404
15.3.7 Examining Accuracy-covariate Associations 406
15.3.7.1 Model Specification Using IPD Studies 407
15.3.7.2 Combining IPD and Aggregate Data 408
15.3.7.3 Application to the Fever Example 408
15.3.8 Performing Sensitivity Analyses and Examining Small-study Effects 409
15.3.9 Reporting and Interpreting Results 409
15.4 IPD Meta-analysis of Test Accuracy at Multiple Thresholds 410
15.4.1 Separate Meta-analysis at Each Threshold 410
15.4.2 Joint Meta-analysis of All Thresholds 410
15.4.2.1 Modelling Using the Multinomial Distribution 411
15.4.2.2 Modelling the Underlying Distribution of the Continuous Test Values 412
15.5 IPD Meta-analysis for Examining a Test’s Clinical Utility 414
15.5.1 Net Benefit and Decision Curves 415
15.5.2 IPD Meta-analysis Models for Summarising Clinical Utility of a Test 416
15.5.3 Application to the Fever Example 417
15.6 Comparing Tests 418
15.6.1 Comparative Test Accuracy Meta-analysis Models 419
15.6.2 Applied Example 420
15.7 Concluding Remarks 420
​
16 IPD Meta-analysis for Prognostic Factor Research 421
Richard D. Riley, Karel G.M. Moons, and Thomas P.A. Debray
16.1 Introduction 422
16.1.1 Problems with Meta-analyses Based on Published Aggregate Data 422
16.1.2 Scope of This Chapter 424
16.2 Potential Advantages of an IPD Meta-analysis 424
16.2.1 Standardise Inclusion Criteria and Definitions 424
16.2.2 Standardise Statistical Analyses 425
16.2.3 Advanced Statistical Modelling 426
16.3 Key Steps Involved in an IPD Meta-analysis of Prognostic Factor Studies 427
16.3.1 Defining the Research Question 427
16.3.1.1 Unadjusted or Adjusted Prognostic Factor Effects? 429
16.3.2 Searching and Selecting Eligible Studies and Datasets 430
16.3.3 Extracting Key Study Characteristics and Information 433
16.3.4 Evaluating Risk of Bias of Eligible Studies 433
16.3.5 Obtaining, Cleaning and Harmonising IPD 433
16.3.6 Undertaking IPD Meta-analysis to Summarise Prognostic Effects 434
16.3.6.1 A Two-stage Approach Assuming a Linear Prognostic Trend 434
16.3.6.2 A Two-stage Approach with Non-linear Trends Using Splines or Polynomials 435
16.3.6.3 Incorporating Measurement Error 438
16.3.6.4 A One-stage Approach 440
16.3.6.5 Checking the Proportional Hazards Assumption 441
16.3.6.6 Dealing with Missing Data and Adjustment Factors 441
16.3.7 Examining Heterogeneity and Performing Sensitivity Analyses 442
16.3.8 Examining Small-study Effects 442
16.3.9 Reporting and Interpreting Results 443
16.4 Software 444
16.5 Concluding Remarks 444
​
17 IPD Meta-analysis for Clinical Prediction Model Research 447
Richard D. Riley, Kym I.E. Snell, Laure Wynants, Valentijn M.T. de Jong, Karel G.M. Moons, and Thomas P.A. Debray
17.1 Introduction 448
17.2 IPD Meta-analysis for Prediction Model Research 448
17.2.1 Types of Prediction Model Research 448
17.2.2 Why IPD Meta-analyses Are Needed 450
17.2.3 Key Steps Involved in an IPD Meta-analysis for Prediction Model Research 452
17.2.3.1 Define the Research Question and PICOTS System 452
17.2.3.2 Identify Relevant Existing Studies and Datasets 452
17.2.3.3 Examine Eligibility and Risk of Bias of IPD 452
17.2.3.4 Obtain, Harmonise and Summarise IPD 454
17.2.3.5 Undertake Meta-analysis and Quantify Heterogeneity 455
17.3 External Validation of an Existing Prediction Model Using IPD Meta-analysis 455
17.3.1 Measures of Predictive Performance in a Single Study 456
17.3.1.1 Overall Measures of Model Fit 456
17.3.1.2 Calibration Plots and Measures 456
17.3.1.3 Discrimination Measures 456
17.3.2 Potential for Heterogeneity in a Model’s Predictive Performance 459
17.3.2.1 Causes of Heterogeneity in Model Performance 460
17.3.2.2 Disentangling Sources of Heterogeneity 461
17.3.3 Statistical Methods for IPD Meta-analysis of Predictive Performance 461
17.3.3.1 Two-stage IPD Meta-analysis 461
17.3.3.2 Example 1: Validation of Prediction Models for Cardiovascular Disease 463
17.3.3.3 Example 2: Meta-analysis of Case-mix Standardised Estimates of Model Performance 466
17.3.3.4 Example 3: Examining Predictive Performance of QRISK2 across Multiple Practices 468
17.3.3.5 One-stage IPD Meta-analysis 469
17.4 Updating and Tailoring of a Prediction Model Using IPD Meta-analysis 470
17.4.1 Example 1: Updating of the Baseline Hazard in a Prognostic Prediction Model 470
17.4.2 Example 2: Multivariate IPD Meta-analysis to Compare Different Model Updating Strategies 471
17.5 Comparison of Multiple Existing Prediction Models Using IPD Meta-analysis 472
17.5.1 Example 1: Comparison of QRISK2 and Framingham 472
17.5.2 Example 2: Comparison of Prediction Models for Pre-eclampsia 476
17.5.3 Comparing Models When Predictors Are Unavailable in Some Studies 476
17.6 Using IPD Meta-analysis to Examine the Added Value of a New Predictor to an Existing Prediction Model 478
17.7 Developing a New Prediction Model Using IPD Meta-analysis 479
17.7.1 Model Development Issues 479
17.7.1.1 Examining and Handling Between-study Heterogeneity in Case-mix Distributions 479
17.7.1.2 One-stage or Two-stage IPD Meta-analysis Models 482
17.7.1.3 Allowing for Between-study Heterogeneity and Inclusion of Study-specific Parameters 483
17.7.1.4 Studies with Different Designs 484
17.7.1.5 Predictor Selection Based on Statistical Significance 484
17.7.1.6 Conditional and Marginal Apparent Performance 485
17.7.1.7 Sample Size, Overfitting and Penalisation 485
17.7.2 Internal-external Cross-validation to Examine Transportability 487
17.7.2.1 Overview of the Method 487
17.7.2.2 Example: Diagnostic Prediction Model for Deep Vein Thrombosis 488
17.8 Examining the Utility of a Prediction Model Using IPD Meta-analysis 491
17.8.1 Example: Net Benefit of a Diagnostic Prediction Model for Ovarian Cancer 492
17.8.1.1 Summary and Predicted Net Benefit of the LR2 Model 493
17.8.1.2 Comparison to Strategies of Treat All or Treat None 493
17.8.2 Decision Curves 493
17.9 Software 494
17.10 Reporting 495
17.11 Concluding Remarks 495
​
18 Dealing with Missing Data in an IPD Meta-analysis 499
Thomas Debray, Kym I.E. Snell, Matteo Quartagno, Shahab Jolani, Karel G.M. Moons, and Richard D. Riley 499
18.1 Introduction 500
18.2 Motivating Example: IPD Meta-analysis Validating Prediction Models for Risk of Preeclampsia in Pregnancy 500
18.3 Types of Missing Data in an IPD Meta-analysis 502
18.4 Recovering Actual Values of Missing Data within IPD 502
18.5 Mechanisms and Patterns of Missing Data in an IPD Meta-analysis 502
18.5.1 Mechanisms of Missing Data 504
18.5.2 Patterns of Missing Data 504
18.5.3 Example: Risk of Pre-eclampsia in Pregnancy 505
18.6 Multiple Imputation to Deal with Missing Data in a Single Study 506
18.6.1 Joint Modelling 506
18.6.2 Fully Conditional Specification 507
18.6.3 How Many Imputations Are Required? 508
18.6.4 Combining Results Obtained from Each Imputed Dataset 508
18.7 Ensuring Congeniality of Imputation and Analysis Models 509
18.8 Dealing with Sporadically Missing Data in an IPD Meta-analysis by Applying Multiple Imputation for Each Study Separately 509
18.8.1 Example: Risk of Pre-eclampsia in Pregnancy 511
18.9 Dealing with Systematically Missing Data in an IPD Meta-analysis Using a Bivariate Metaanalysis of Partially and Fully Adjusted Results 511
18.10 Dealing with Both Sporadically and Systematically Missing Data in an IPD Meta-analysis Using Multilevel Modelling 514
18.10.1 Motivating Example: Prognostic Factors for Short-term Mortality in Acute Heart Failure 515
18.10.2 Multilevel Joint Modelling 516
18.10.3 Multilevel Fully Conditional Specification 519
18.11 Comparison of Methods and Recommendations 521
18.11.1 Multilevel FCS versus Joint Model Approaches 521
18.11.2 Sensitivity Analyses and Reporting 523
18.12 Software 523
18.13 Concluding Remarks 524
Part V References 525
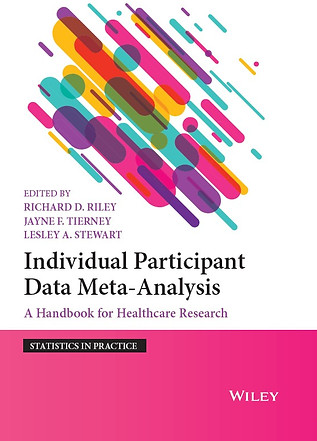